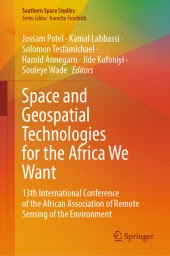
Lead Scientist, Dr. Lisa Rebelo, recently contributed a paper to "Space and Geospatial Technologies for the Africa We Want" - a book compiled from the outcomes of the 13th International Conference of the African Association of Remote Sensing of the Environment (AARSE) international conference.
The paper deals with the recognition of the importance of land cover data, and the role of EO data to provide these. However, problems of access, storage, pre-processing and analysis of EO data limit its use and uptake, and it was shown how the platform and datasets of Digital Earth Africa can overcome these. This paper follows on from a recent collaboration between Digital Earth Africa and the United Nations Food and Agriculture Organization (FAO) EOSTAT Field Boundary Digitizing Mapathon.
The FAO launched the Earth Observation for Official Statistics (EOSTAT) project to support the Hand-in-Hand (HiH) initiative. EOSTAT aims to utilise innovative approaches, diverse data sources, and advanced analytical tools to drive agricultural progress. Currently, there are insufficient resources for the regular collection, analysis, and dissemination of agricultural statistics. Earth Observation (EO) data presents a perfect solution to address this gap, enhancing the ability to generate reliable crop statistics at both national and subnational levels.

As part of this initiative, FAO has partnered with Digital Earth Africa and FrontierSI to create an open-source, user-friendly platform that will help African nations map land cover and crop types. A key achievement of this collaboration has been the development of a prototype land cover classification workflow within the Digital Earth Africa platform, designed to be scalable for monitoring land use at various scales, from local to national. An initial land cover map for Rwanda has been created, with the results validated through comparison with validation data and engagement with local stakeholders.
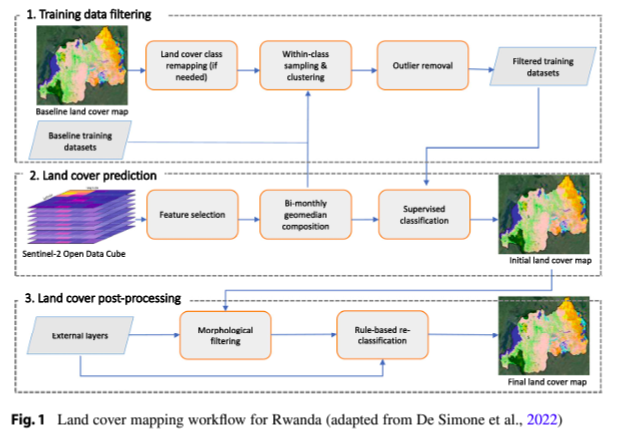
The land cover mapping workflow was built within the DE Africa Sandbox, a user-friendly environment offering a wide range of Python libraries and packages. The Sandbox allows users direct access to analysis-ready satellite imagery, making it easier for users, even those without advanced technical expertise, to conduct detailed analysis and gain insights into land use and crop classification. Previous concerns around data storage are alleviated with the cloud storage of extensive Digital Earth Africa datasets.